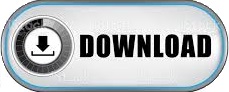
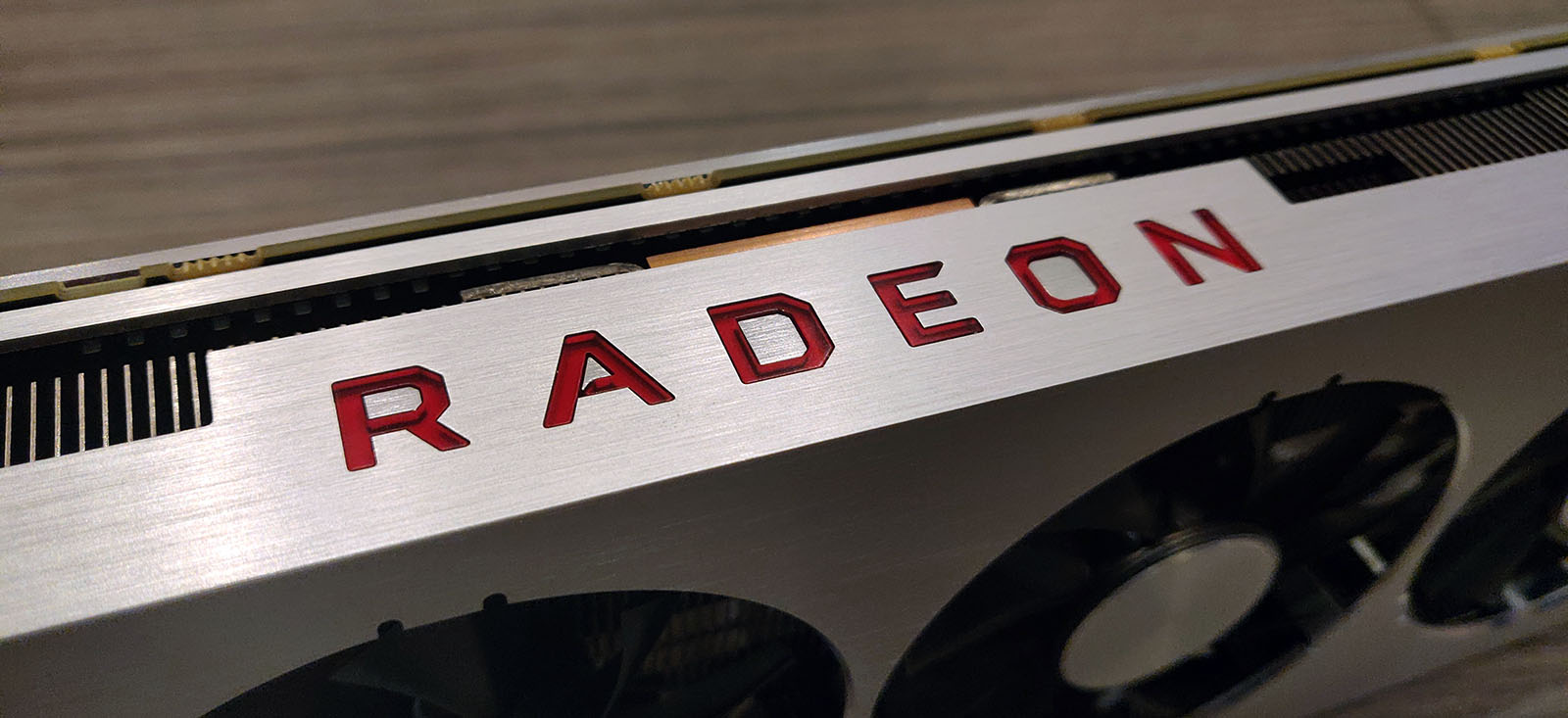
Some of Nvidia’s customers apparently wanted a part that fit in a much smaller thermal envelope yet still had a decent price and acceptable performance for inference – hence the A2. The Nvidia A2 accelerator announced during the GTC 2021 fall conference last week actually makes use of a very cut-down GA107 GPU, which has not been used in datacenter or edge use cases thus far. We did a deep dive on the GA102 and its accelerators and graphics card uses in October 2020.
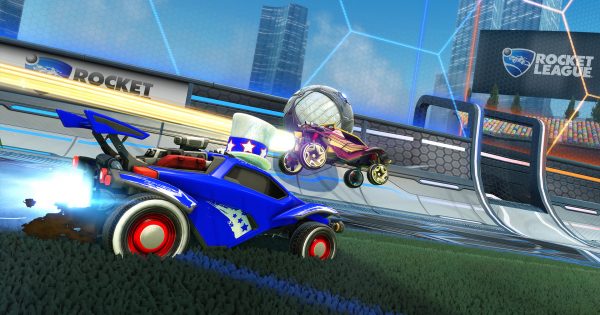
The GA102 GPU, which was predominantly aimed at gaming uses, also supports inference and visualization (thanks to RT ray tracing cores), and is deployed in the Nvidia A10, A40, and A6000 accelerators. The GA100 is designed specifically for dense datacenter compute – the kind that drives HPC simulation and modeling as well as AI training workloads. We focus on the Nvidia compute engines suitable for datacenter and edge uses here at The Next Platform, and that has meant the GA100 variant of the Ampere architecture used in the big, bad A100 accelerator launched in May 2020 as well as the A30 accelerator that came out in April 2021. It is better suited to hyperscale and cloud datacenters with modest machine learning inference workloads as well as edge computing workloads where low power, low cost, and reasonable performance are all imperative. Nvidia has pulled these levers in a slightly different way to come up with an inference engine variant of its “Ampere” GA107 GPU, called the A2 and etched in 8 nanometer processes from foundry partner Samsung. Even in a world dominated by X86 general purpose compute, the SKU stacks still got pretty hairy, and by comparison, even the ever-widening “Ampere” GPU accelerator product line from Nvidia is still relatively simple.īut, to attract different customers, it still takes optimizations of price, thermals, and performance.
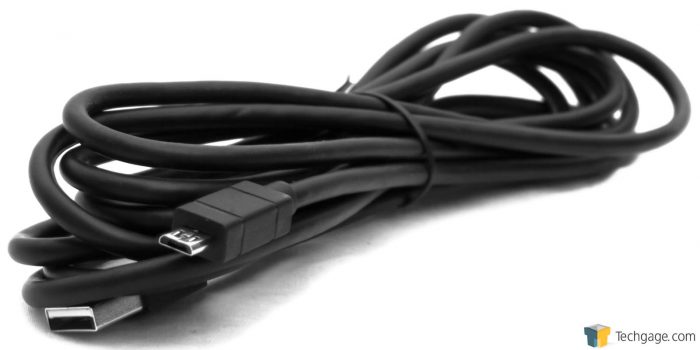
The world would be a simpler place for all processing engine makers if they just had to make one device to cover all use cases, thus maximizing volumes and minimizing per unit costs.
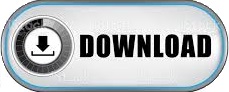